In the era of digital transformation, Artificial Intelligence (AI) can redefine the way companies operate, innovate, and interact with customers. Generative AI models empower organizations to create new and unique content, ranging from images and text to voices, with remarkable accuracy and relevance.
Customizing AI to meet a company’s specific goals is a process that extends beyond the technology itself, encompassing organizational culture, operations, and business strategy. The path to developing and implementing customized AI presents challenges and overcoming them requires a structured approach.
This article discusses the nature, evolution, and impacts of Generative AI Models in the business environment, highlighting how customizing these tools can become a competitive advantage.
What are Generative AI Models?
Generative AI Models are a type of artificial intelligence technology focused on creating new content, whether it be texts, images, music, or any other type of data that can be digitally simulated. These models learn the characteristics of training data to generate new examples that, while original, resemble the original data. They are fundamental in a variety of applications, including:
- Text Creation: Generate coherent and contextually relevant texts by learning from examples of human texts. They can be used to create articles, reports, and more.
- Image Generation: Create realistic images of objects, people, landscapes, or even artworks that never existed, based on learning from a large number of real images.
- Voice and Music Synthesis: Capable of generating human voice or music that sounds natural, learning from voice recordings or music.
Generative models are an area of active research and rapid evolution in AI, with growing applications across various fields.
The Evolution of Custom Generative AI in Business
Generative Artificial Intelligence is revolutionizing the way companies operate, boosting innovation, automating tasks, creating unique content, and offering customized solutions on a large scale. As the technology evolves, customizing generative AI tools and applications has enabled solutions more aligned with the specific needs of each business. This evolution reflects not only technological advancements but also a significant change in companies’ approach to integrating AI into their operations.
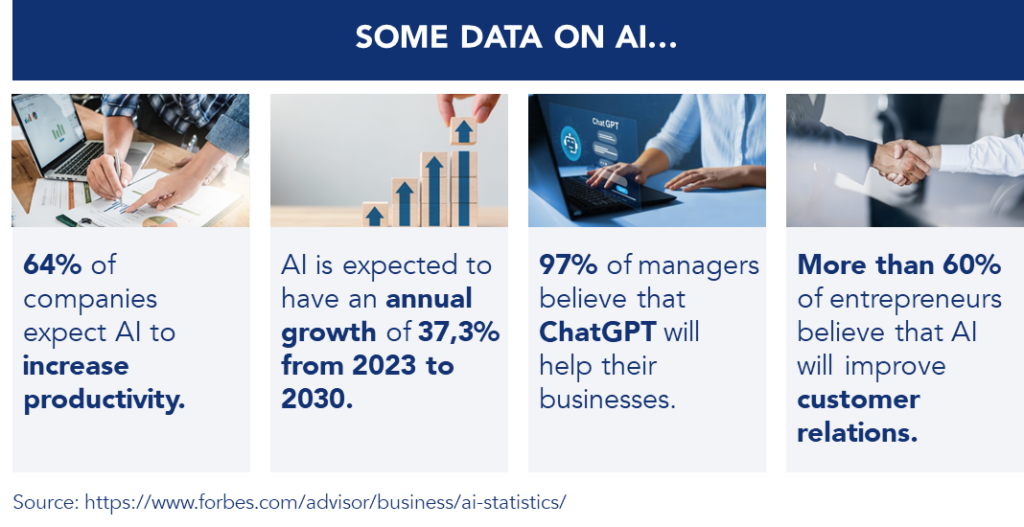
Understanding the Shift to Custom AI Solutions
Generic models of generative artificial intelligence, such as ChatGPT, have captured the attention of a broad audience. However, in practice, customized models can provide greater value to organizations.
The shift towards customized AI solutions is driven by an increasing understanding that generic tools often cannot meet the specific needs of each company. Customizing Generative AI involves adjusting models for specific tasks, integrating with existing systems, and taking into account unique data sets that reflect the particularities of each business. This approach offers significant advantages, such as improved operational efficiency, greater accuracy in forecasting and analysis, and the ability to generate highly relevant content for specific audiences.
Weighing Open Source Versus Proprietary Models for Business
When adopting customized Generative AI, companies face the decision between open-source and proprietary models. Each option has its advantages and disadvantages.
Open-Source Models
Advantages: Access to cutting-edge technologies without significant license costs, flexibility to customize and adapt the code to specific needs, and an active community that contributes to improvements and support.
Challenges: Requires a qualified technical team for implementation and maintenance, there may be less formal support available, and the responsibility for security and compliance rests with the company.
Proprietary Models
Advantages: Generally, offer a more polished and user-friendly platform, with dedicated customer support. The service provider takes responsibility for security, updates, and compliance, which can reduce the burden on the company’s internal resources.
Challenges: The cost can be a barrier, especially for small and medium-sized businesses. Customization and integration with existing systems can be limited compared to open-source solutions. Additionally, dependence on a specific supplier can present long-term risks.
The decision between open-source and proprietary models should be based on a careful assessment of the company’s specific needs, available resources, and long-term goals. While open-source solutions may offer greater flexibility and control, proprietary models can provide a more streamlined and secure solution for implementing Generative AI.
Tailoring AI to Fit Enterprise Objectives
Implementing Artificial Intelligence in business processes is becoming a strategic necessity across various industries. However, to extract maximum value from AI’s capabilities, it’s crucial that companies not only adopt this technology but also tailor it to align with their specific goals. Customizing and adjusting AI solutions according to the unique needs of an organization can unlock unknown potential, optimize operations, and boost innovation.
Advantages of Fine-Tuning AI with Enterprise Data
Customizing AI using company-specific data brings significant advantages. AI models adjusted with internal data tend to offer more accurate and relevant results. This happens because the models are trained with the company’s data, allowing them to recognize patterns and trends unique to the organization. On the other hand, using internal data allows companies to maintain control over their sensitive information, reducing the risk of data exposure, which can occur when relying on external data sources or pre-trained models.
Achieving Business-Specific Outcomes with Custom Models
Customizing AI models allows companies to achieve specific results, as it enables solutions to be aligned with the strategic objectives of the business.
Customized models are developed with clear objectives, meaning every aspect of the model, from data selection to learning framework, is optimized to achieve specific outcomes. Customized AI models can be developed to explore new growth areas, create innovative products or services, and improve the customer experience.
Adapting AI to meet the company’s specific goals is a continuous process of alignment between technology and strategy. This effort not only ensures that AI implementations are more effective but also ensures that AI-driven innovations are deeply integrated into the organization’s vision and goals. As AI continues to evolve, the ability to customize and adjust these technologies will be a competitive differentiator for companies looking to lead in their sectors.
Challenges of Developing Custom AI
Developing customized Artificial Intelligence solutions presents a promising path for companies looking to optimize their operations. However, this process is not without challenges. Organizations need to overcome a series of technical, operational, and strategic obstacles in order to successfully implement customized AI.
Identifying Roadblocks in Custom AI Development
The process of developing customized AI involves several stages, from the conception of the idea to implementation and continuous improvement. Along this path, companies face obstacles in various areas, with some of the most critical being data quality, integration with existing systems, and the acquisition of suitable talent.
Data quality, integration, and talent challenges
Data quality is the backbone of the success of AI projects. Inaccurate, incomplete, or biased data can lead to AI models that are ineffective, generate erroneous results, or perpetuate prejudices. Companies must:
- Data Cleaning and Preparation: Invest time and resources in cleaning and preparation to ensure that data sets are complete, accurate, and free of bias.
- Data Governance: Establish robust data management policies to maintain data quality over time and ensure compliance with privacy and data protection regulations.
Integrating customized AI solutions with existing business systems and processes requires:
- Technology Compatibility: Ensure that AI solutions are compatible with the existing IT infrastructure, which may require updates or modifications to current systems.
- Data Flow: Establish an efficient data flow between systems to feed AI models with up-to-date data and capture AI-generated insights into business operations.
- Organizational Change: Manage change within the organization, ensuring that employees adapt to the new tools and processes introduced by AI.
Acquiring and developing talent with the necessary skills to create, implement, and maintain customized AI solutions is another challenge. The high demand for AI professionals can make it difficult to hire qualified talent, so companies should proceed with:
- Hiring, Training and Development: Invest in continuous training and development for internal teams, ensuring they can keep up with rapid advances in AI technology. It is also important to hire new AI professionals, if necessary.
- Multidisciplinary Collaboration: Foster effective collaboration with multidisciplinary teams, including data specialists, software engineers, and project managers, to ensure the development of holistic and well-integrated solutions.
Overcoming these challenges requires a structured and focused approach. Companies that invest in data quality, effective integration of AI solutions, and building capable teams will be better positioned to develop and implement customized AI that can positively impact the business.
Benefits of custom enterprise generative AI models
Companies face several challenges with the generative AI models available on the market, including a disconnection from the specific business needs, data privacy risks, complexity in integrating these models with the company’s current systems, and difficulty in scalability. Customized generative AI models, when tailored to the specific needs and objectives of an organization, can provide significant competitive advantages. Here are some of the main benefits that these models offer to businesses:
- Improved Quality of Generated Content: Customized models, when trained with company-specific data, produce more relevant and accurate content, from detailed analyses to marketing material that resonates with the target audience.
- Operational Efficiency: Customization allows for the automation of repetitive tasks, increasing productivity and minimizing human error. This is applicable in various areas, from customer service to supply chain management. The integration of OpEx and AI can bring significant results for organizations.
- Innovation in Product Development: Customized models can reveal valuable insights through data analysis, indicating opportunities for new products or improvements to existing ones.
- Personalization at Scale: Custom models enable the creation of personalized experiences at scale, from product recommendations to specific marketing content, positively impacting customer satisfaction and loyalty.
- More Informed Decisions: These models generate predictive analytics and insights from vast data sets, assisting in the prediction of market trends and consumer behavior, facilitating more accurate decision-making in volatile environments.
- Security: Designed with a focus on data protection, customized AI models ensure that privacy and security of information are maintained, reducing the risks associated with data management.
- Ease of Integration and Scalability: Customized AI models generally simplify integration with existing systems, allowing for more agile implementation and efficient scaling as business needs evolve, ensuring that technology grows in parallel with the company.

The journey of customized generative AI is just beginning. As technology advances, more organizations are expected to adopt these customized solutions to remain competitive and achieve their strategic goals. To fully obtain the potential of these technologies, it’s crucial that leaders are able to manage and mitigate risks, develop the right skills in their professionals, and adapt existing processes, managing changes efficiently.
Still have some questions about Custom enterprise gen AI models?
What is custom AI?
Customized AI refers to the development and application of artificial intelligence systems that are tailored or customized to meet the specific needs, goals, and challenges of a company. These systems use specific data, business objectives, and user preferences to provide more relevant and effective solutions.
What is generative AI?
Generative AI is a subfield of artificial intelligence focused on creating models that can generate new data similar to the data on which they were trained on. These models are capable of producing original content, such as images, text, music, and even code, which mimics the distribution of the original data, enabling a wide range of creative and analytical applications.
What are the two main types of generative AI models?
The two main types of generative AI models are:
Generative Adversarial Networks (GANs): A system composed of two neural networks, one generative and one discriminative, which compete with each other to generate increasingly realistic data.
Variational Autoencoders (VAEs): Work by encoding the input data into a lower-dimensional representation (latent coding) and then decoding that representation to reconstruct the input data. During the learning process, the model learns to generate new data by decoding random samples of the latent space.
See more on Digital & AI
Find out more about improving your organization